Understanding Customer Behavior Through Data
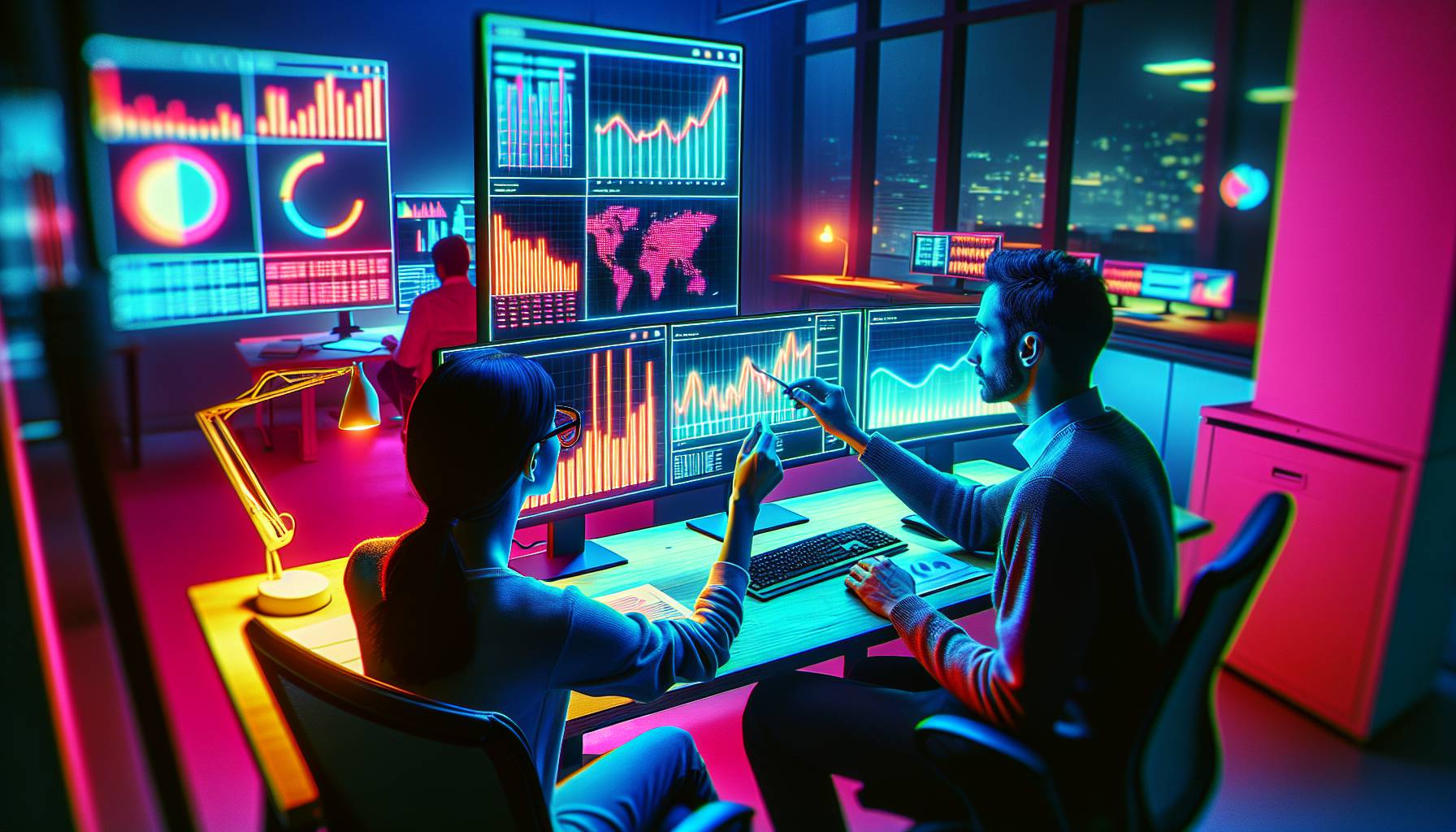
Are you looking to deepen your understanding of customer behavior through data analytics? If so, you’re in the right place. In today’s competitive e-commerce landscape, grasping how customers interact with your brand is crucial for driving sales and improving overall business performance. This article will guide you through key data insights that reveal valuable patterns in customer behavior, helping you make informed decisions that can enhance your sales strategies.
Understanding customer behavior through data is essential for tailoring your marketing efforts and improving customer experiences. By analyzing various touchpoints, such as website visits, purchase history, and social media interactions, you can gain insights into what motivates your customers to make a purchase. This understanding allows you to create personalized marketing campaigns, optimize your product offerings, and ultimately increase conversion rates.
As you dive into the data, consider how segmentation can play a role in your analysis. By grouping customers based on demographics, purchasing habits, or preferences, you can identify trends that may not be apparent when looking at aggregate data. This targeted approach enables you to cater to specific customer needs and enhance their shopping experience, leading to higher customer satisfaction and loyalty.
Moreover, leveraging behavioral analytics tools can provide real-time insights into customer interactions, allowing you to make quick adjustments to your strategies. For instance, if you notice a high abandonment rate in your shopping cart, you can investigate further to understand the underlying causes and implement solutions, such as retargeting campaigns or improved checkout processes.
In summary, this article will equip you with six essential data insights that will empower you to harness analytics effectively for better sales. By understanding customer behavior through data, you will be well on your way to optimizing your e-commerce strategy and achieving your business goals.
Identifying Sales Trends and Patterns
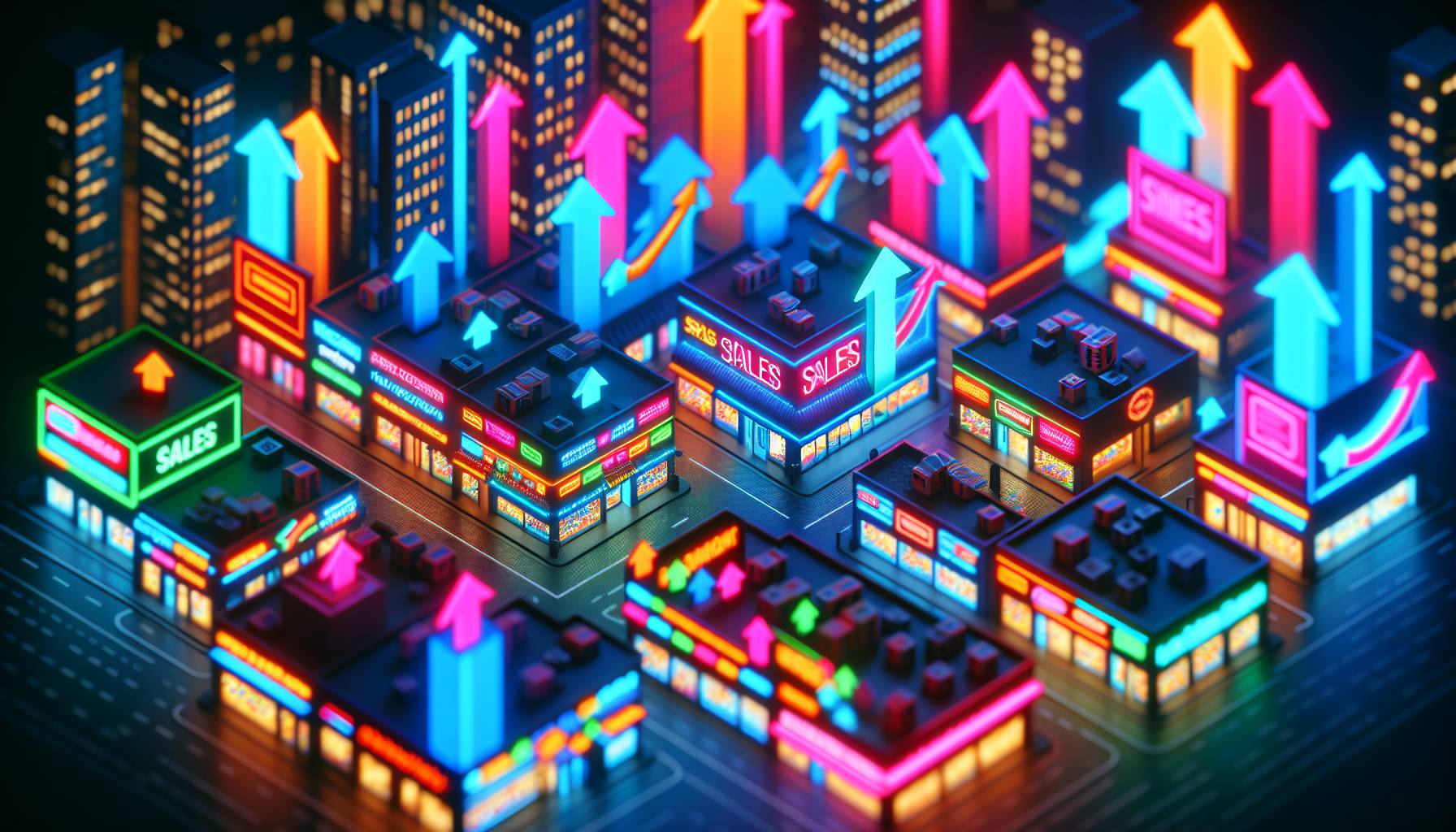
To effectively boost sales, one must dive deep into the data to unearth trends and patterns that can inform strategic decisions. This process begins by gathering historical sales data, which can reveal fluctuations based on various factors such as seasonality, market demands, and customer preferences. By analyzing this data, businesses can identify peak sales periods, allowing them to tailor their marketing efforts and inventory management accordingly.
Another crucial aspect of recognizing sales trends is segmenting data by demographics, geographic location, and purchase behavior. This segmentation enables companies to pinpoint which customer groups are driving sales and which products resonate most with specific demographics. For instance, a retailer may discover that a particular product line performs exceptionally well in urban areas but lags in rural markets. Such insights can guide targeted advertising campaigns and promotional efforts that cater to specific audience preferences.
Utilizing advanced analytics tools, businesses can also leverage predictive analytics to forecast future sales trends based on historical data. This technique involves using statistical algorithms and machine learning to predict customer behavior, enabling companies to proactively adjust their strategies. For example, if data indicates a growing trend in eco-friendly products, a company may decide to expand its offerings in that category before the demand peaks, thereby positioning itself advantageously in the market.
Furthermore, regular analysis of sales data should be incorporated into the decision-making process. By establishing a routine, businesses can stay ahead of market changes and consumer preferences. This could involve monthly or quarterly reviews of sales performance, allowing teams to quickly adapt to any emerging trends or shifts in the market landscape.
Lastly, engaging with sales staff and collecting their insights can complement data analysis. Frontline employees often have a unique perspective on customer interactions and can provide valuable qualitative data that may not be captured by analytics tools. Combining quantitative data with qualitative insights creates a comprehensive view of sales trends, leading to more informed decisions and strategies.
Leveraging Predictive Analytics for Sales Forecasting
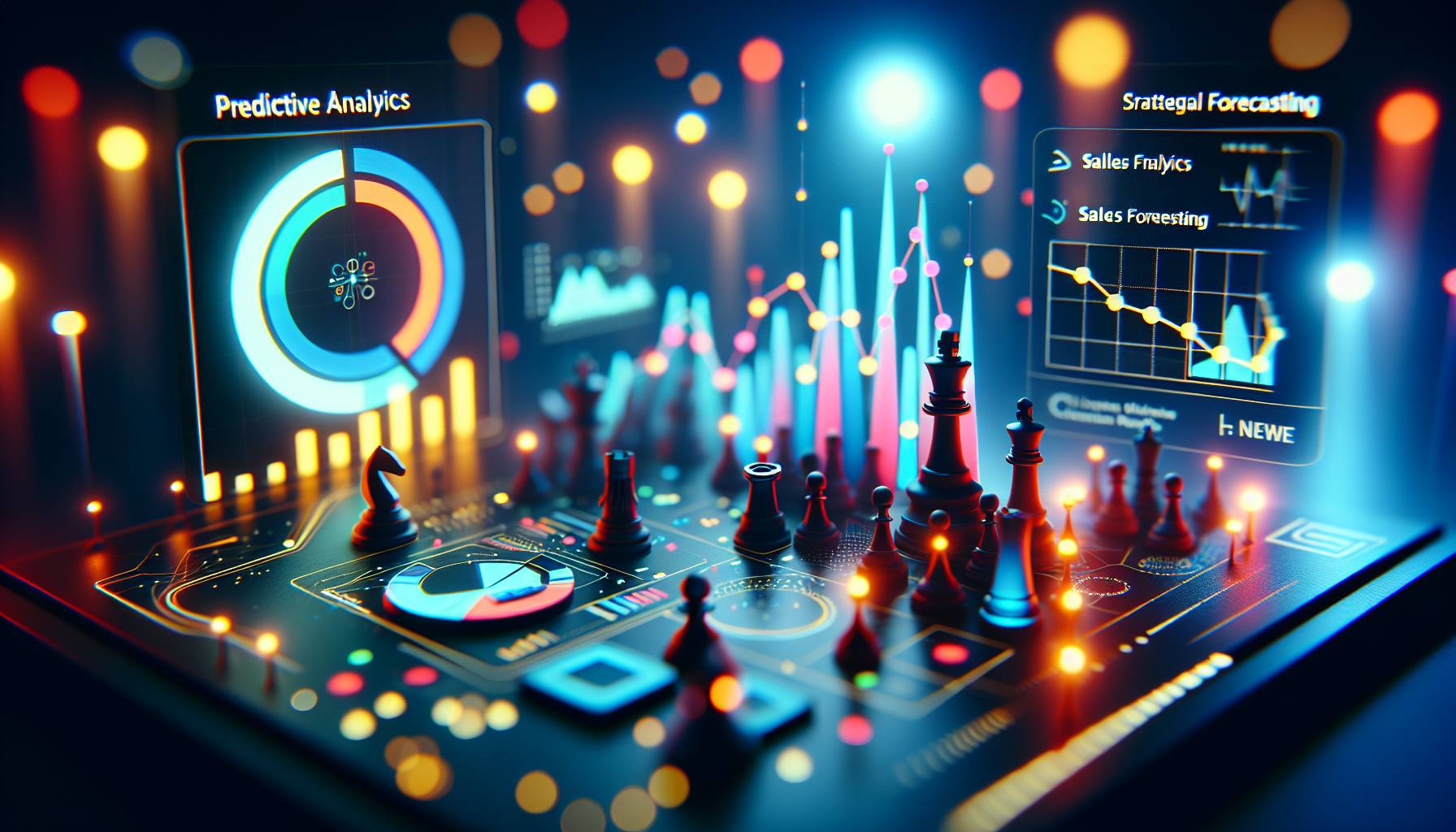
Predictive analytics has emerged as a powerful tool for businesses looking to enhance their sales forecasting accuracy. By utilizing historical data, statistical algorithms, and machine learning techniques, companies can identify patterns and trends that inform future sales outcomes. This proactive approach allows organizations to anticipate market demands, optimize inventory levels, and allocate resources more effectively.
To effectively leverage predictive analytics in sales forecasting, companies should start by gathering and integrating data from various sources, including CRM systems, sales transactions, and market trends. By creating a comprehensive dataset, businesses can gain insights into customer behavior, seasonality, and economic indicators that influence sales. Once the data is collected, advanced analytics tools can be employed to analyze these variables, generating forecasts that are not only based on past performance but also on predictive models tailored to the specific business context.
Another crucial aspect of employing predictive analytics is the continuous refinement of forecasting models. As new data becomes available, organizations should regularly update their models to ensure accuracy. This iterative process allows businesses to adapt to changing market conditions and customer preferences swiftly. Moreover, by incorporating real-time data feeds, such as social media sentiment or competitor pricing, sales forecasts can become even more responsive and accurate.
Collaboration between sales teams and data analysts is also vital in this process. Sales professionals possess valuable insights into customer interactions and preferences that can enhance the predictive models. By fostering a culture of data-driven decision-making, organizations can ensure that their sales strategies are aligned with the most current and relevant information available.
Ultimately, the integration of predictive analytics into sales forecasting not only improves accuracy but also empowers organizations to make informed strategic decisions. By anticipating future sales trends, businesses can proactively address challenges, seize opportunities, and drive growth in an increasingly competitive landscape.
Enhancing Customer Segmentation with Data Insights
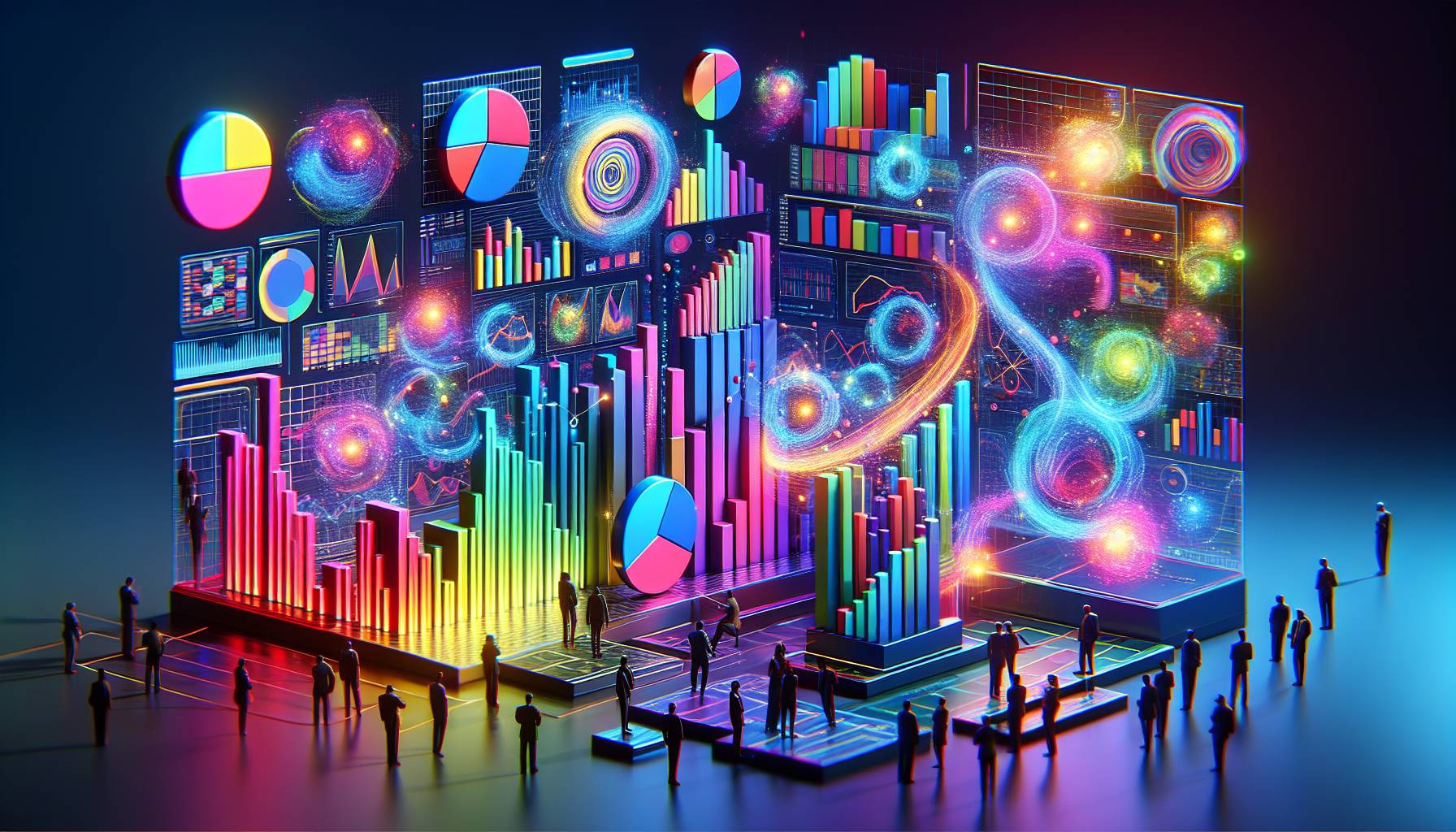
In the realm of sales, understanding your customers is key to tailoring your offerings and maximizing engagement. By leveraging data insights, businesses can refine their customer segmentation strategies, allowing for more targeted marketing and improved customer experiences. The process begins with collecting diverse data points, such as demographic information, purchasing behavior, and online interactions. Analyzing this data helps identify distinct customer groups based on shared characteristics and preferences.
Once these segments are established, the next step involves deeper analysis to uncover trends and patterns within each group. For instance, advanced analytics can reveal which products are favored by specific segments, how often they purchase, and their preferred communication channels. With this information, companies can create tailored marketing campaigns that resonate more effectively with each segment, increasing the likelihood of conversion and customer loyalty.
Moreover, data insights can enhance predictive analytics capabilities, allowing businesses to anticipate customer needs and behaviors. By utilizing historical data and machine learning algorithms, companies can predict future buying patterns within each segment. This foresight enables proactive marketing strategies, such as personalized offers and timely promotions, ensuring that businesses stay relevant and appealing to their target audience.
Additionally, continuous monitoring and analysis of customer feedback and engagement metrics can provide real-time insights that further refine segmentation efforts. A/B testing different approaches on various segments can yield valuable data about what resonates best, leading to ongoing optimization of marketing strategies. This iterative process not only improves customer satisfaction but also drives higher conversion rates and sales growth.
Ultimately, enhancing customer segmentation through data insights empowers businesses to forge stronger connections with their audience. By understanding and anticipating customer needs, companies can deliver personalized experiences that foster loyalty and drive revenue, solidifying their competitive advantage in an ever-evolving marketplace.
Optimizing Pricing Strategies Using Analytics
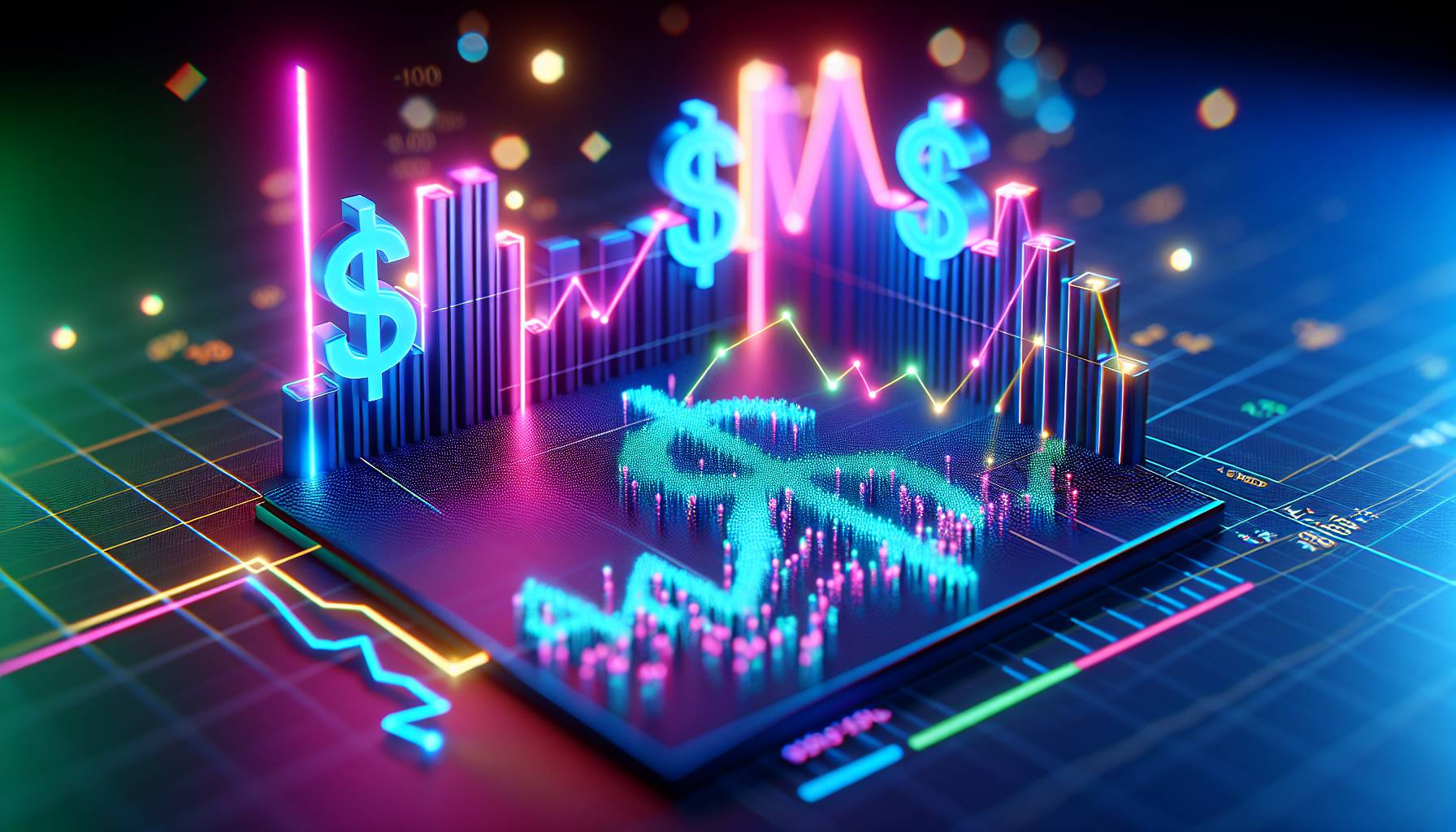
One of the most impactful applications of analytics in sales is in the realm of pricing strategies. By leveraging data analytics, businesses can gain deep insights into customer behavior, market trends, and competitive positioning, allowing them to set prices that maximize both sales volume and profit margins. Understanding the elasticity of demand is crucial; analytics can help identify how sensitive customers are to price changes. This knowledge enables businesses to implement dynamic pricing strategies, adjusting prices in real-time based on market conditions, customer demand, and inventory levels.
Another key component of pricing optimization is segmenting customers based on their willingness to pay. By analyzing historical data, businesses can identify different customer segments and tailor pricing strategies accordingly. For instance, premium customers may respond well to value-based pricing, while price-sensitive segments might require promotional offers or discounts to encourage purchases. Advanced analytics allow for the creation of personalized pricing models, increasing customer satisfaction and loyalty while enhancing profitability.
Moreover, predictive analytics can forecast the likely impact of pricing changes on sales performance. By simulating various pricing scenarios, organizations can determine the optimal price point that balances revenue and demand. This not only aids in setting initial prices but also informs future pricing adjustments based on seasonal trends, economic factors, or shifts in consumer preferences. Through continuous monitoring and analysis, businesses can remain agile and responsive to market changes, ensuring their pricing strategies are always aligned with business goals.
Finally, integrating analytics with other business functions, such as marketing and supply chain management, enables a holistic approach to pricing. For example, marketing campaigns can be tailored to promote products at strategic price points, while supply chain insights can inform decisions on inventory levels to support pricing strategies. By fostering collaboration across departments and utilizing a data-driven approach, companies can optimize their pricing strategies effectively, leading to improved sales performance and customer engagement.
Measuring the Impact of Marketing Campaigns on Sales
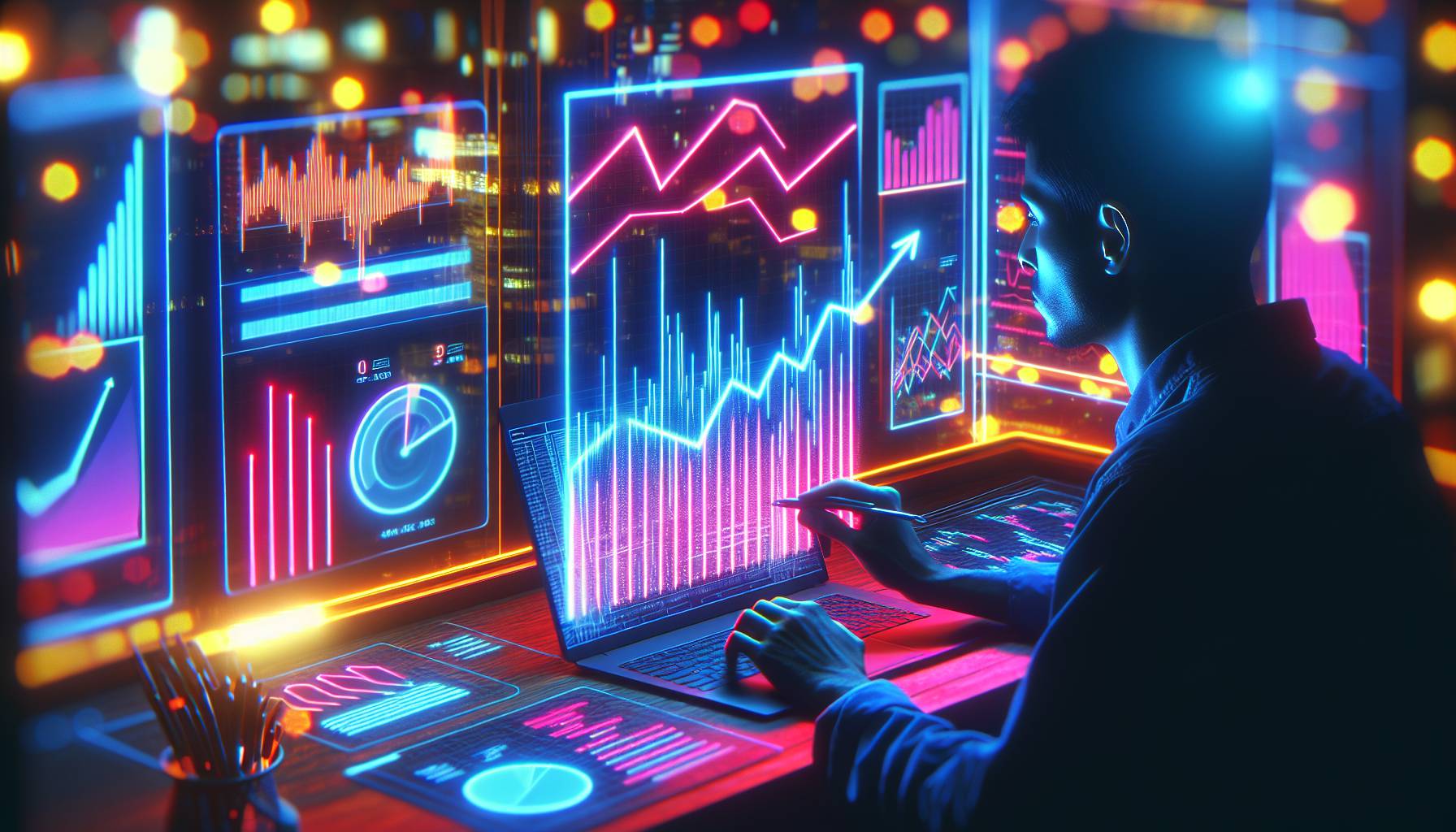
Understanding the effectiveness of marketing initiatives is crucial for any business aiming to optimize its sales performance. By analyzing how marketing campaigns directly influence sales outcomes, organizations can make informed decisions about where to allocate resources and how to refine their strategies for greater impact.
One of the most effective ways to assess the impact of marketing efforts is through the use of key performance indicators (KPIs). Metrics such as conversion rates, return on investment (ROI), and customer acquisition costs provide valuable insights into how well a campaign is performing. By establishing clear KPIs before launching a campaign, businesses can create benchmarks that will help measure success post-campaign.
Another valuable approach is to implement A/B testing. This method involves running two variations of a marketing campaign simultaneously to determine which performs better. By comparing the sales generated from each variant, businesses can identify the most effective messaging, visuals, or calls to action. This data-driven decision-making process allows companies to refine their marketing strategies for future campaigns.
Attribution modeling plays a critical role in understanding how various touchpoints in the customer journey contribute to sales. Businesses can utilize multi-touch attribution models to analyze how different marketing channels work together to drive conversions. This helps in recognizing the cumulative effect of various campaigns, enabling marketers to allocate budget more effectively across channels.
Collecting customer feedback post-purchase is also an essential tactic for measuring campaign success. Surveys and reviews can provide insights into how customers perceived the marketing messages and whether they influenced their buying decisions. This qualitative data complements quantitative sales figures and gives a fuller picture of a campaign's effectiveness.
Finally, integrating marketing analytics tools allows for real-time tracking of campaign performance. These tools can provide instant feedback on various metrics, making it easier to adjust campaigns on the fly and optimize them for better results. By continuously monitoring performance, businesses can pivot strategies as needed to enhance their impact on sales.
In summary, measuring the outcomes of marketing efforts on sales is a multi-faceted process that involves defining KPIs, employing A/B testing, utilizing attribution models, gathering customer feedback, and leveraging analytics tools. By adopting these strategies, businesses can gain actionable insights that drive improved performance and ultimately lead to increased sales success.